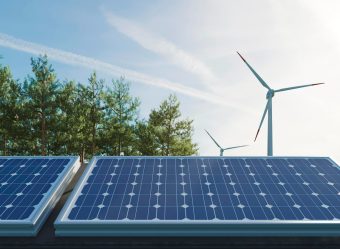
Power systems are becoming vastly more complex as demand for electricity grows and decarbonisation efforts ramp up. In the past, grids directed energy from centralised power stations. Now, power systems increasingly need to support multi-directional flows of electricity between distributed generators, the grid and users. The rising number of grid-connected devices, from electric vehicle (EV) charging stations to residential solar installations, makes flows less predictable. Meanwhile, links are deepening between the power system and the transportation, industry, building and industrial sectors. The result is a vastly greater need for information exchange – and more powerful tools to plan and operate power systems as they keep evolving.
This need arrives just as the capabilities of artificial intelligence (AI) applications are rapidly progressing. As machine learning models have become more advanced, the computational power required to develop them has doubled every five to six months since 2010. AI models can now reliably provide language or image recognition, transform audio sounds into analysable data, power chatbots and automate simple tasks. AI mimics aspects of human intelligence by analysing data and inputs – generating outputs more quickly and at greater volume than a human operator could. Some AI algorithms are even able to self-programme and modify their own code.
More:
- ABB INVESTS IN STRATEGIC PARTNERSHIP WITH CLEAN ENERGY START-UP TO OFFER END-TO-END WIND ENERGY PORTFOLIO
- IRENA GLOBAL ATLAS UPGRADE ENHANCES UNDERSTANDING OF COUNTRIES’ RENEWABLES POTENTIAL
- RENEWABLES JOBS NEARLY DOUBLED IN PAST DECADE, SOARED TO 13.7 MILLION IN 2022
It is therefore unsurprising that the energy sector is taking early steps to harness the power of AI to boost efficiency and accelerate innovation. The technology is uniquely placed to support the simultaneous growth of smart grids and the massive quantities of data they generate. Smart meters produce and send several thousand times more data points to utilities than their analogue predecessors. New devices for monitoring grid power flows funnel more than an order of magnitude more data to operators than the technologies they are replacing. And the global fleet of wind turbines is estimated to produce more than 400 billion data points per year.
This volume is a key reason energy firms see AI as an increasingly critical resource. A recent estimate suggests that AI already serves more than 50 different uses in the energy system, and that the market for the technology in the sector could be worth up to USD 13 billion.
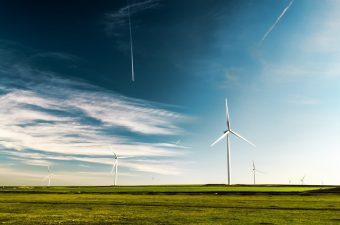
One of the most common uses for AI by the energy sector has been to improve predictions of supply and demand. Developing a greater understanding of both when renewable power is available and when it’s needed is crucial for next-generation power systems. Yet this can be complicated for renewable technologies, since the sun doesn’t always shine, and the wind doesn’t always blow.
That’s where machine learning can play a role. It can help match variable supply with rising and falling demand – maximising the financial value of renewable energy and allowing it to be integrated more easily into the grid.
Wind power output, for example, can be forecast using weather models and information on the location of turbines. However, deviations in wind flow can lead to output levels that are higher or lower than expected, pushing up operational costs. To address this, Google and its AI subsidiary DeepMind developed a neural network in 2019 to increase the accuracy of forecasts for its 700 MW renewable fleet. Based on historical data, the network developed a model to predict future output up to 36 hours in advance with much greater accuracy than was previously possible.
This greater visibility allows Google to sell its power in advance, rather than in real time. The company has stated that this, along with other AI-facilitated efficiencies, has increased the financial value of its wind power by 20 percent. Higher prices also improve the business case for wind power and can drive further investment in renewables. Notably, Google’s proprietary software is now being piloted by a major energy company.
Additionally, with a more accurate picture of peaks in output, companies like Google are able to shift the timing of peak consumption, such as during heavy computing loads, to coincide with them. Doing so avoids the need to buy additional power from the market. This capacity, if expanded more widely, could have a significant impact on the promotion of load shifting and peak shaving – especially if combined with better demand forecasts. For example, Swiss manufacturer ABB has developed an AI-enabled energy demand forecasting application that allows commercial building managers to avoid peak charges and benefit from time-of-use tariffs.
Eead the full text here
Source: IEA